AIREVOLUTIONIZES PATHOLOGY
DR MONA JAMWAL
DR POONAM SHARMA
DR REETIKA MENIA
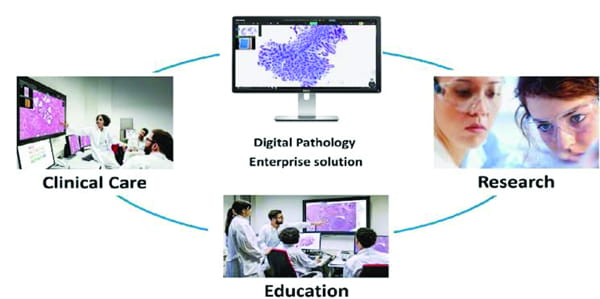
John McCarthy introduced the term “Artificial intelligence” in 1956, while Arthur Samuel coined “machine learning” in 1959, defining it as the ability to learn autonomously. Artificial intelligence (AI) is increasingly becoming important in the field of medicine. The term AI refers to the intelligence presented by some non-human artificial entities, including computers and robots in pathology.
Role of AI in Pathology: AI and machine learning technologies are enhancing efficiency and accuracy in diagnosing diseases like cancer. Digital pathology, which uses advanced imaging and AI algorithms, is becoming more common, providing faster and more precise diagnoses. With AI’s ability to analyze complex data effectively, it is helping improve precision medicine for cancer patients. Automated whole slide imaging scanners now produce high-quality images of tissue samples, which, combined with digital pathology tools, streamline the diagnostic process across different areas of Pathology. Notably, in 2018, the FDA sanctioned the first medical device utilizing AI for detecting diabetic retinopathy in adults. Subsequently, in 2021, the FDA authorized the first AI-based software, Paige Prostate, for detecting prostate cancer. Machine learning (ML) techniques, a subset of artificial intelligence (AI), are increasingly employed in pathology to automate image analysis tasks and boost diagnostic precision. These methods encompass a broad spectrum of functions, from basic object recognition to intricate pattern analysis for forecasting disease diagnosis, prognosis, and treatment outcomes. By extracting image patches and utilizing deep learning algorithms, AI enables in-depth spatial examination of histological features, resulting in quicker and more dependable diagnoses. Additionally, AI streamlines tasks, allowing pathologists to focus on complex cases and meet growing workload demands. It identifies regions of interest, prioritizes cases, and introduces novel staging paradigms, enhancing workflow efficiency. Deep learning, a cornerstone of AI and ML, employs neural networks to process data and make predictions, offering significant potential for advancing diagnostic capabilities in pathology.
AI in pathology has dual applications: clinically, it streamlines tasks like cell counting and cancer detection, enhancing accuracy and saving time for pathologists. Moreover, it acts as a quality control tool, boosting confidence in diagnostic reports. In research, AI delves into complex medical data, uncovering novel disease patterns crucial for tailored treatments and prognoses. For instance, it accurately predicts renal cancer prognosis, surpassing traditional methods. AI also excels in forecasting therapy response in colorectal cancer patients and identifying biomarkers in digital slide images, heralding a pivotal role in pathology’s evolution and therapeutic advancement.
AI Applications in Diagnostics: Recent advancements in AI have revolutionized cancer diagnosis and classification, particularly within pathology. Integrated into diagnostic workflows, AI tools tackle various tasks like object recognition and segmentation, aiding pathologists in discerning complex information. For instance, AI enhances sensitivity by efficiently detecting isolated tumor cells in lymph nodes suspected of metastasis. It also standardizes scoring criteria in tumors like prostate and breast cancers, where morphology indicates biological processes. Content-based image retrieval (CBIR) empowers pathologists to search vast databases for images resembling specific cases, crucial for diagnosing rare and complex conditions accurately and promptly. This technology highlights similarities in histopathological features, facilitating diagnoses in challenging cases beyond visual resemblance.
Predictive and Prognostic Applications of AI: AI shows promise in predicting prognosis and treatment responses based on histological features, offering vital insights efficiently. By correlating images with tumor characteristics, microenvironment, and genetic profiles, AI can provide concise forecasts for survival outcomes and therapy outcomes. While integrating diverse morphological features into a unified prognostic index presents challenges for humans, AI-based image analysis offers a novel classification system. This system depicts clinical outcomes, recurrence likelihood, and therapeutic response by associating key histological features like tumor morphology and stromal architecture. Deep learning methods within computational pathology (CPATH) unearth distinctive biomarkers, such as tumor-infiltrating lymphocytes (TILs), whose spatial distributions hold diagnostic and prognostic value. TILs, crucial in anticancer immunity activation, could serve as reliable biomarkers if objectively quantified across the tumor microenvironment (TME).
AI as a Predictor of Molecular and Genomic Profiles: AI tools play a crucial role in extracting insights into tumor genetics and genomic profiles from morphology, aiding in understanding cancer biology. Molecular-based tests, like the mRNA-based oncotype test, offer prognostic information by combining various parameters. While linking morphological patterns to tumor genetics seems straightforward, integrating vast genomic data, such as next-generation sequencing (NGS), presents challenges. Although combining imaging and molecular features can provide a comprehensive view of tumors, developing, training, and validating models to handle such complex multidimensional data remain significant challenges.
The Role of AI in Research, Training, and Education: AI tools are invaluable resources for enhancing pathologists’ training, offering interactive features and annotations to create dynamic learning environments. This integration enriches morphology knowledge with advanced technologies, enabling personalized and precision medicine practices. Already utilized in teaching settings like conferences and virtual workshops, AI tools, alongside clinical and research registries and sophisticated laboratory information systems, provide comprehensive support in pathology practice. By integrating AI into daily workflows, trainees receive supplementary information for differential diagnoses and diagnostic skill refinement, enhancing resident training and collaboration between institutions for efficient consultations on challenging cases.
Future Directions and Opportunities: In recent years, the development of AI tools for cancer detection by various companies has surged, with the FDA’s approval of the Philips whole-slide scanner in 2017 marking a significant milestone in clinical workflow digitization. The COVID-19 pandemic prompted some institutions to adopt digital workflows, despite the challenges involved. Despite obstacles, there’s been a notable shift in digital pathology, with the introduction of open-top light sheet microscopy offering non-destructive, slide-less 3D tissue images, potentially enhancing AI applications with greater spatial and architectural information. While current AI can identify tumor scores and grades, future applications may likely remain focused on narrow AI tasks.
In the coming decade, AI tools are expected to become commonplace in pathology labs, with a high likelihood of being utilized for a wide range of tasks. These tasks include identifying micro metastases and lymph node metastases, quantifying various stains, counting mitoses and lymphocytes, and automating quality control processes for slides and images. Additionally, AI is anticipated to play a significant role in prioritizing cases, standardizing pathology reports, and assisting with laboratory workflow management. In recent years, novel AI approaches in pathology have shown significant potential to improve diagnostic workflows, reduce errors, and enhance prognostic predictions. However, challenges such as interpretability, validation, regulation, and cost have slowed their integration into clinical practice. To meet the growing demand for personalized cancer care, integrating AI with human pathologists and existing systems could be beneficial. A multimodal approach combining proteomics, genomics, and AI-based biomarker quantifications may be crucial for precise tumor therapy.
(The authors Dr. Mona Jamwal is Senior Resident, Dr. Poonam Sharma is Associate Professor and Dr. Reetika Menia is Assistant Professor, Department of Pathology, AIIMS Jammu).